AI Training Data 101 Guide: Foundation Data
- BCMstrategy, Inc.
- Jan 4
- 5 min read
2025 is the year when data buyers and vendors become serious and discerning regarding AI training data. The proliferation of quantitative and language data used to train a range of AI models for expanding use cases may also generate confusion as buyers attempt to distinguish among different kinds of data.
And so we start 2025 by providing an AI Training Data 101 Guide. This series of three posts describes the main kinds of data: Foundation Data (volume, velocity), Derivative Data (volatility, momentum, correlation, averages), and Synthetic Data. Today's post focuses on Foundation Data
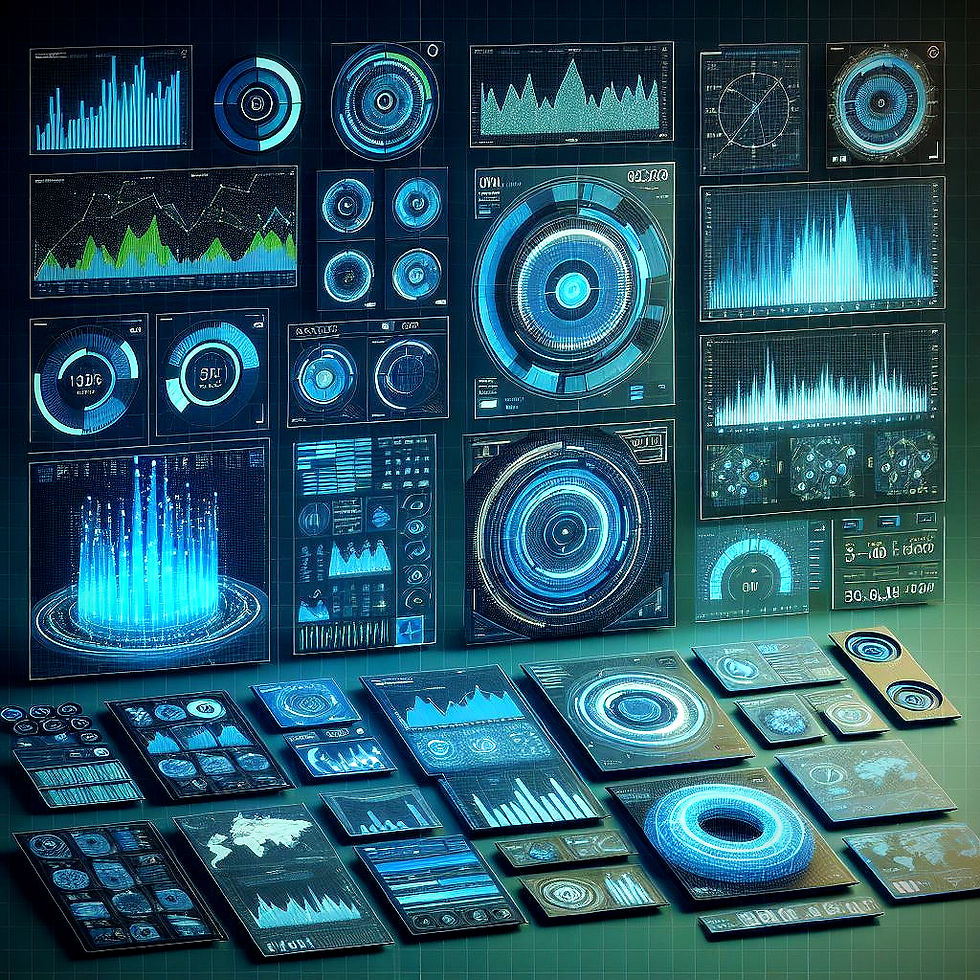
Background -- The AI Training Data Context
AI models at their core provide unparalleled pattern matching capabilities. Both quantitative AI models and generative AI models spot patterns within input datasets with speed and accuracy rates that humans cannot match. The capacity to spot correlations and repeatable patterns drives predictive analytics farther along the innovation frontier. But it is not magic.
The strategic consequence for economic activity is profound: significant jumps in productivity. Freed from the drudgery of pattern matching activities, humans allocate time and intellectual capital to analyzing context and meaning, identifying implications, and anticipating outcomes.
The ability to extract productivity gains and informational advantages from AI models thus requires a laser-like focus on ensuring that the models have the correct and best data inputs.
It's harder than it sounds. Advances in technology made it possible to measure more kinds of units. AI model outputs themselves create derived data.
Welcome to your 2025 AI Training Data 101 Guide
Foundation Data -- Volume and Velocity
Foundation data consists of components that form the crucial underpinning for analysis. Foundation data is always objective. It delivers veracity (observable facts) by measuring two key units: volume and velocity.
AI models (both quantitative and generative AI) use foundation data to deliver accurate aggregations across multiple categories of data.
A growing array of measurement devices makes observable a broader range of activities that previously had been undetectable. This includes "exhaust data" generated from smart devices (e.g., smart watches, smart automobiles) as well as human language.
Volume Data; Measures units within a specific time period.

Traditional examples: temperature, physical amounts, stock trading prices by the millisecond; manufacturing production line outputs by the hour or day; carbon emissions by the hour; kilowatts of energy produced by the hour or day.
21st Century examples: bytes of data/memory, language token size, bits per character; entropy (amount of information produced by a language model on a per-letter basis, expressed in relation of the average number of binary digits per letter, word error rates), the distance between nodes in a knowledge graph.
Velocity Data; Measures speed across time for items that move.
Traditional examples: miles per hour for vehicles; cycle times for processes
21st Century examples; Processor Hertz (clock cycles per second); bits per second (data transmission speeds); FLOPs (floating point numbers measuring computational effort needed to process data within a defined period of time)
Traditionally, volume and velocity data could only be measured in relation to physical objects. Language technology makes it possible to measure volume and velocity also in relation to words.
For example, our patented, award-winning PolicyScope process makes it possible to measure the volume and velocity of public policy language daily and automatically in order to identify which policy issues are most in play on any given day in any given country. The quantification mechanism operates in addition to, and separate from, word counting.
Yes, it IS possible to measure volume and velocity in public policy. We do it every day, subject to an award-winning, patented process that we authored.
The bottom line: advanced technology illuminates and makes measurable a wider range of observable activity to support analytical activity. When delivered to AI processes, the data supports faster pattern identification at scale which increases analytical productivity exponentially.
However, humans still need to define the inputs...which means data buyers need to become more savvy about which specific data that can deliver volume and velocity measurements. AI processes by themselves at least initially do not know which measures are the most useful for analytical measurement. If a data buyer does not know that a particular volume or velocity measurement can be captured, they will never request it. Sophisticated data scouts during 2025 will become more intrepid about finding new kinds of data that extend analytical advances and operational efficiencies; in some cases, they may even educate internal audiences about newly discovered data points.
Data buyers will also be taking a deeper dive into the backgrounds of the data vendors. It takes deep domain expertise both to identify what kind of volume and velocity measurements deliver meaningful insights and to craft appropriate mechanisms for capturing the measurements consistently, automatically, and objectively. Just because a firm has fancy technology does NOT mean that the team has the domain expertise to find and create appropriate datasets.
Foundation Language Data for Foundation Language Models
Foundation language data involves far more than just assembling input files consisting of raw text. Language processing is very compute-intensive. A kitchen-sink approach to language input files translates into highly expensive as machines climb the learning curve associated with the language data inputs. While some costs have begun to decrease in the last few months, the process of acquiring and sifting through language inputs remains highly resource intensive, which is inefficient and prone to errors on the output side.

Compiling robust, effective, and objective language data inputs regarding public policy creates considerable additional challenges.
Engineers must attempt to control for inherent bias both in the collection process and inside the language data.
Technology experts rarely if ever know where to find accurate, reliable, high quality language data inputs.
Taking a 'kitchen sink' approach to data collection may be quick, but time and capital will be required to support multiple training runs as the models attempt to sort out context.
Even when text has been enriched with metadata tags at the source, those tags may not align with a firm's preferred ontology or they may be too high-level to be useful.
Hallucination risk is high. This is language that moves markets and changes peoples' lives. Processing official sector text through models trained on consumer chatbot, movie review, and social media posts creates considerable risks for the technology to generate wildly incorrect and misleading outcomes.
Foundation language data solves for these problems and inefficiencies.
BCMstrategy, Inc. is the first company to take a disciplined and intentional approach to compiling and structuring official sector language data for use as the foundation for generative AI-powered research applications.
Our deep expertise in public policy means we know where policy signals appear, making our compilation/input process far more efficient from the beginning.
Our deep subject matter expertise and our deep expertise in quantitative data for modeling means that we can deliver the language structured objectively with an ontology expressed as both quantitative and conceptual metadata tags that increase the efficiency of deployment of the language into knowledge graphs that support retrieval-augmented generation processes.
For firms preferring off-the-shelf solutions, we can also deliver tokenized embeddings or dedicated research AI agents powered by generative AI trained on our structured language data.
Conclusion
Competitive pressures to achieve productivity gains by deploying AI-powered trend projection and generative AI-powered research during 2025 will increase the need for firms to make more intentional choices about their training data. Applying a consistent rubric both to quantitative and language data will enable firms to identify value quickly and accurately, starting with volume and velocity data.
The next post in this AI Training Data 101 series will address derivative or analytical data. The third post will address synthetic data.
BCMstrategy, Inc. generates a broad range of AI training data from the language of public policy. We generate multivariate time series data, tickerized to major currencies and the Russell 3000, in order to support AI-powered predictive analytics and quantitative policy trend projection. We also generate generative AI training data in a broad range of formats to support advanced research and analysis regarding climate/energy policy, digital currency and tokenization policy, and global macro policy (e.g., monetary policy, de-dollarization trends, and trade policy).